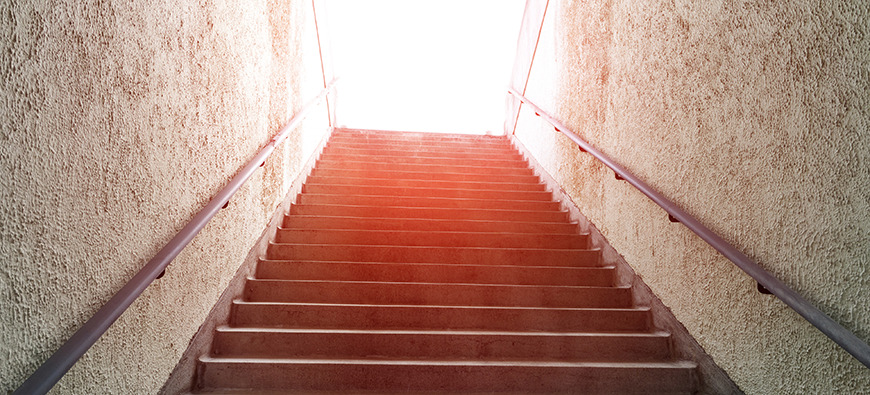
The Next Step in FICO Credit Modeling
Brought to you by Bankers Healthcare Group
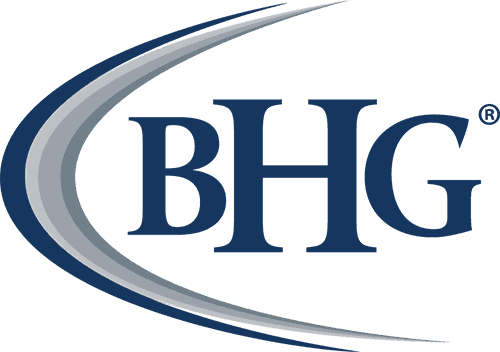
Since its introduction by Fair, Isaac & Co. more than three decades ago, FICO has long been considered the No. 1 standalone credit decisioning model. Is there a way the banking industry can build on that foundation to create a more future-forward way of predicting lending outcomes?
The way the financial services industry analyzes data has evolved since FICO’s inception in 1989. New and exciting technology has led to innovativeu202falgorithms that give bankers a more defined look at an even greater data set. An all-encompassing view of a borrower’s story can bring a new realization: these new methods of analyzing credit, combined with the FICO mainstay, can lead to even better outcomes for everyone.u202fu202f
Many facets make up an individual’s credit story – beyond payment history and amounts owed. There is data that, once analyzed, can give lenders critical insights into borrower characteristics that can’t be categorized by a single number. Peopleu202fare more dynamicu202fthan their credit scores.u202fu202f
Imagine a traditional consumer credit scoring model as a printed picture: a one-dimensional take on a person’s whole life in credit. In that static picture, there are balances on debt obligations, utilization of revolving types of credit like credit cards, delinquency and statuses, among others. This information comes from the three major credit bureaus – Equifax, TransUnion and Experian – and represents a vast cross-section of loans originated by banks, credit unions, finance companies and other lenders across the credit industry.u202fThisu202finformation adds up to that singleu202fdefinitiveu202fscore.
In contrast, non-traditional models that build on the foundation of FICOu202fcan incorporateu202fadditional predictive information.u202fThink of it as theu202fmotion picture version that creates a more dynamic view of consumer creditworthiness.u202fThis modelu202fgives lenders an ability to assess point-in-time information and the momentum of trended credit data factors, which may help predict the future credit conditions for a potential borrower and allow a lender to make more informed decisions.u202fBankers have greater visibility into the depth of a borrower’s story, like balances or utilization increasing or decreasing, and can capture that relationship with risk outcomes.
Alternative data sources can complement static and trended credit history by introducing consumers’ checking history, property ownership and alternative finance activity into credit scoring models.u202fConsumers with comparable credit files can have vastly different repayment history and patterns; incremental information related to creditworthiness equips lenders to optimize risk differentiation when the credit file alone doesn’t capture the full story.
Creating a new model tou202fcalculateu202fandu202fpredict high-performing loans is no small feat. BHG Financial, a leader in unsecured business and personal loans and creator of one of the country’s largest community bank loan networks,u202fonceu202frelied on the traditional credit scoring model to help with their decision-making. The company decided to evolveu202ftheir credit model to identify miscategorized but high-quality borrowers that most lenders were missing.
BHGu202fFinancialu202fdata scientists partnered with TransUnion to analyze over 2 million consumer loans; each loan was over $20,000, had at least 36-month terms and originated between 2015 and 2017. This amounted to more than a billion pieces of data points to analyze and assess, resulting in their proprietaryu202fcredit model, theu202frScore.u202f An updated credit model resulted in faster approvals, and the identification of subprime borrowers that perform well along with prime borrowers with high default rates.
Evolving the already successful and established FICO score, the chances are lower that good-paying borrowers will be labeled as high risk. This enables some lenders to approve pockets of creditworthy consumers that others might decline. At the same time, the chance of labeling risky borrowers as low risk also declines – allowing lenders to protect the credit quality of their portfolio.
Lenders unable to dedicate time and money to develop their own evolved credit scoring model can collaborate with companies that have created updated credit models, skipping the extensive research and the costly origination process. This gives them immediate access to purchasing top-quality loans with low risk, which can quickly strengthen their loan portfolio to meet their bank’s criteria. This solutionu202fisu202fpossibly the best answer to finding a moreu202ffuture-forward way of predicting lending outcomes.u202fu202fu202fu202f